AI Objectives - AIMS ISO 42001 Framework
The ISO 42001 standard has some key objectives, which are supposed to guide organizations towards using and developing AI Technology in a sustainable way. This might remind you of the sustainability objectives set in the ESG taxonomy.
The key AI Objectives of ISO 42001 based AIMS are:
- Accountability
- Environmental Impact
- Fairness
- Privacy
- Maintainability
- Robustness
- Safety
- Security
- Transparency
- Explainability
- AI Governance
- Reliability
- Accessibility
In this article we will look at every objective in detail as it is important to understand them properly. Designing a customized AIMS requires that you addresss the above mentioned objectives in a way that matches your business modell and activities.
AI Objectives according to ISO IEC 42001
The international Standard ISO IEC 42001:2022 has a range of objectives. They are driven by many issues evolving from the way AI has been used in the past. Governments, civil rights advocators and industry leaders want to put AI onto sustainable tracks. The following objects are introducted in relation with the observed issues and desired to rectify the situation.
Accountability
The ISO 42001 standard requires organizations to become more accountable when using AI technology. Hence, an AIMS must provide the management foundation for an accountability framework. Too often unregulated AI models cause damages to members of the public. By addressing accountability inside the AIMS, an organization will take measures to counter potential operational hazards, legal concerns, and brand damage. When writing the AIMS it is necessary to list the key stakeholders which will be users, their companies, AI developers, AI vendors, training data providers and regulatory bodies.
Environmental Impact
AI systems have a considerable environmental impact, due to their higher energy consumption and use of natural resources. Hence, their ecological footprint influenced by the way AI technologies are developed, deployed, and run. Key aspects to consider are energy consumption by data centres during model training, circular economy of hardware, carbon footprint, obsolescence and e-waste.
Fairness
AI is often blaimed for wrong decions due to an inherent bias. Fairness in AI is aimed at countering algorithmic bias. Automated decision processes using machine learning models may result in biased or unfair outcomes. Organizations need to address bias in order to ensure sensitive variables doesn’t lead to bias where machine-learning decisions are taking place. Sensitive variables are information on a person’s gender, race, sexuality, or disabilities.
Such adverse biases can lure inside model at various stages:
1. Business problem formulation:
If the business problem is not properly defined and its usage has not borders, then fairness will definately be compromised. Make sure to define the use case clearly, set well described limitations and exceptions, and name those people who are accountable in developing the AI-ML model.
2. Training Data:
During the training of AI and data validation, problems might start evolving without being noticed. This will lead to undesired results due to unfairness inherent in the data used for training.
- Bias in sample data: The training dataset might not representative and lead to misinterpretations. Here one must review the dataset for issues in sampling, presence of hidden proxy variables, legal or consent issues. Such issues can lead to racial, gender or political bias.
- Identifying and transforming sensitive features: If a sample contains a disfavoured group, then the model weights must be adjusted. The outcome for this disfavoured group should then be acceptable.
3. Training Algorithm / model architecture:
Biases can be introduced as the design’s result of the model. It is important to handle this issue as it can result in misleading results. Such adverse outcome will compromise an outcome even when using good data.
- Model Build: One must be aware that model architecture suffers from inherent problems which cause bias: Regression bias, Classification bias or Clustering bias. Calculation errors can occur in model parameters. This results in overfitted/underfitted models. Eventually bias and noise compromisse the output data.
- Model Drift: As AI-ML models are fed with data and used for automated decisions, issues will flow back into the dataset and increase the problematic situation. Thereby, the business problem will change its character. This makes it necessary to re-modell and re-train. Invitably this will lead to re-introducing biases into the AI System.
Fortunately, fairness can be measured with the help of specially designed mathematical models. Here, statistical independence, confidence intervals, separation study and sufficiency study assist in this measring of the level of fairness.
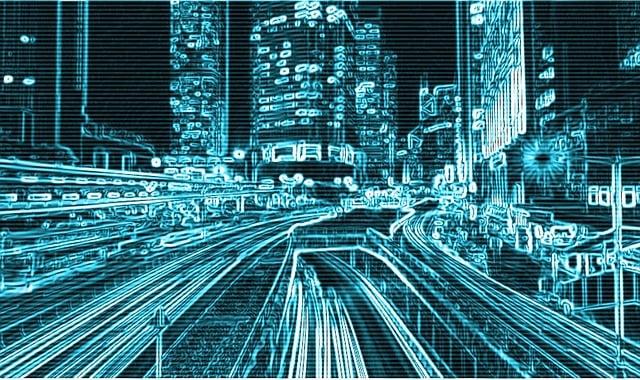
Privacy
AI technology processes a large amount of data. The source of such data is sometimes highly questionable as it may result in breach of confidentiality, privacy violations or even intellectual property violations. The way data is processed is an additional zone of conflict. Hence, privacy and data protection issues arise. People might be harmed as a result of unauthorized disclosure of sensitive data. The misuse of such data is inacceptable. Trends show that malicious actors have acquired sensitive data, by adversarial attacks and model poisoning. This enables them to manipulate AI-driven choices, or impair AI system integrity and reliability. As a result, privacy and security are severly compromised.
Mass surveillance and privacy concerns are driven by the widespread adoption of AI-powered facial recognition and location tracking technology for fighting crime. A person’s habits, actions, and movements become fully tracable. Such data can be misued by governements and cyber criminals. Hence, it violates privacy rights and civil freedoms. This where ISO 42001 and the EU AI Act are trying to positively guide AI technology towards a more socially safe design. Such privacy by design in AI needs to become part of the lifecycle, data anonymization and minimization in aI projects. It is important to prove compliance with rules and standards can solve such problems.
Maintainability
Organization must show their efforts at addressing the problems of their AI usage. Where necessary, AI systems will have to be adapted to meet new requirements. Hence, maintainability shows the organization’s ability to readjust the AI systems:
- Modularity and reusability: By applying a modular design of AI systems, it is possible to reuse/replace components without affecting the entire AI system.
- Documentation and version control: The documentation of AI projects must be comprehensive and consistant. Items such as code comments and user manuals should be up-to-date. The documentation of data preprocessing, model training and deployment processes may not display significant gaps. Version control systems are not new to developers. They make tracking changes, collaborating with others, and reverting to previous versions much easier.
- Code quality: When coding for AI projects, it is necessary to maintain a Code Hygiene. High-quality code must follow accepted best practices. Proper naming conventions, consistent style, and efficient algorithms reduce developer frustration and improve maintenance cost.
- Scalability: The AI system’s design should allow a scale up or down as needed. Performance and resource management should remain balanced in what ever direction scaling is taking place.
Robustness
AI Robustness requires AI technology to be resilient to input data or model parameter changes. Hence, the AI Robustness will ensure consistent and reliable performance even in unexpected scenarios. Robustness provides reliability and resilience of the model to dynamic changes in the data it is using. Unfortunately, this is not a standalone solution to AI’s issues. Overly conservative models decrease fairness of the applied models.
Model robustness is as follows:
- Data pipeline: where data validation modules check for peculiarities in future data
- Model pipeline: where it is ensured that the model can’t be attacked to produce undesirable outputs
- System robustness: AI models will be part of applications. The entire pipeline should be secure.
Evaluating the robustness of AI systems using quantitative metrics allow you to observe, how well the models perform under different stress conditions. Such conditions may include adversarial attacks, noise, and data distribution shifts. False positives/negatives rate, MSE study, Wasserstein distance and Brier score can be used as key metrics.
Safety
Safe AI Systems should not endanger human life, health, property, or the environment. Our expectations towards the safety of AI system sis even greater where autonomous vehicles or healthcare robotocs can costs lives should the systems dangerously malfunction. Hence, rigorous testing and validation must ensure AI Systems meet safety standards and regulatory requirements. Introducing multiple safegards by providing fail-safes and redundancies, should prevent failures from causing harm or significant disruption.
The idea of a Human in the Loop (HITL) desired to enhance AI system safety. Critical decision-making scenarios may overwhelme an AI system and thereby require humans to intervene, override, or guide the AI’s actions where necessary.
Security
Security in AI systems should protect the AI models, data and infrastructure against threats and vulnerabilities. The confidentiality, integrity and availability of the system and its data are paramount.
Data Security is there to prevent data used by AI system from being tampered with. It should safegard it against unauthorized access.
Model security needs to protect AI models from being reverse-engineered or poisoned by unauthorized parties. The model and data has to be defended against adversarial attacks. Malicious actors want to manipulate inputs to mislead the model. There is a range of ways to mitigate such attacks: adversarial training, input validation, anomaly detection, access control, audit and monitoring, risk assessment and patch management.
Transparency
According to ISO 42001 AI transparency provides insights into the data AI system uses and how it makes decisions. This allows us to understand, why the AI system act in the particular way. The organisation and the users are the two perspectives of transparency. Organisation perspective refers to the people who are responsible of decision making, maintaining models and data streams. In contrast, user perspective knows the data origin and form as well as how the organization uses it.
Transparency has 3 levels:
- Algorithmic transparency (logic and model)
- Interaction transparency (user interface)
- Social transparency (impact of this interaction on society)
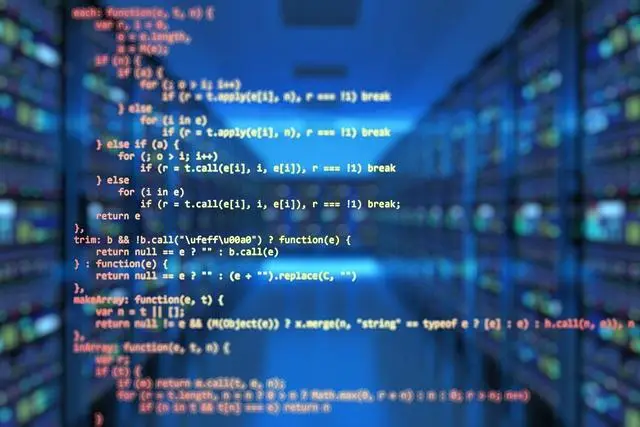
Explainability
The complexity of AI-ML technologies are increasingly difficult for the public to understand. This increasingly leads to anxiety and distrust due to a lack of understandable explainations. This is where explainability allows AI models to be understood by humans. The decision-making process can thereby be tracked in a more human-friendly manner. Organisations have to understand the models from both technical and business standpoint. Explainability allows organisations to building confidence in the AI-ML models. Tools like LIME and SHAP allow model explainability to be measured. The idea is to analyse the input-output predictions by the models.
AI Governance
It is important to consider the ethical implications of integrating human components into models and applications. Technology may not harm society or users. It is in the interest of AI developers, users and legislators to ensure that AI technologies are developed and used in accordance with society’s values. Concerns regarding bias, privacy, and misuse must be addressed. Promoting innovation and trust is only possible when AI Governance is capable of handling misuse.
Reliability
Stakeholders expect AI technology to be reliable and deliver valid AI outputs. Outputs have a direct influence on effective decisions. It is paramount to uphold stakeholders’ trust in the organization. AI systems with no or limited human input can minimize risks to a better extent. In order to deliver consistent outcomes and maintain performance excellence, there are a few key items to do: they need to be trained, validated, monitored, transparent, and undergo continuous improvement.
Accessibility
A wide range of people are accessing AI technology. There are professional AI Developers and those who are playing around for a wide range of reasons. Some people are designing more user-friendly interfaces. Entry barriers to AI are often language diversity and accessibility for individuals with disabilities. In developing countries the cost of AI technology is often a financial and energy related issue. Hence, we see individuals with diverse abilities and needs interacting with AI. User empowerment wants to help users gain access to the tools and information required for taking advantage of AI related opportunities. Making informed decisions about AI interactions build trust and increases the adoption rate of this new technology. Education and transparency are the key foundations to user empowerment around the world. Users want to understand, how AI systems work and how to control them. A positive and inclusive AI experience for all users benefits all ethical stakeholders.
Conclusion
By handling the AI Objectives and being compliant with ISO 42001 an Organisation has an opportunitiy to become compliant with EU AI Regulation. Developing the policies and procedures are not an easy task. There are not enough well proven AIMS templates on the market, as consultants have to develop the documentation.